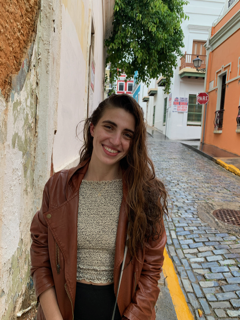
Jennifer Dawkins, HST MEMP PhD student
The 2022 Ralph G. (1970) and Claire Brindis Family Fellow uses machine learning to incorporate prior scientific knowledge into datasets and models
HST
When Jennifer “Jen” Dawkins came to MIT for the Harvard-MIT Program in Health Sciences and Technology (HST), she was particularly eager to combine her knowledge of machine learning with an enduring interest in medicine.
Harvard’s Gerber Lab, which works to develop advanced computational methods to solve biomedical problems, was an ideal choice. Jen’s research—as part of HST’s Medical Engineering and Medical Physics (MEMP) PhD program—is supported by the Ralph G. (1970) and Claire Brindis Family fellowship. It involves modeling the recurrence of C. difficile infections in the gut from microbiome samples.
Jen says the Gerber Lab is such a good fit because its research creates a “strong connection to the medical side of things while working on very interesting computational problems.”
Praise for the working relationship in the lab goes both ways. Gerber, (an HST MD and MEMP PhD graduate; Assistant Professor of Pathology at Brigham and Women's Hospital and Harvard Medical School) says one of the remarkable things about Jen is the way she exemplifies the HST MEMP mindset by speaking three "languages" fluently: life sciences, medicine, and computer science.
"Jennifer is able to move between deciphering complex biochemical transformations, figuring out their clinical significance, developing the theory for sophisticated new machine learning models, and programming in Python—all in the same day," Gerber says.
Working with medical data presents a unique challenge relative to most other data: namely, it is much more difficult and expensive to obtain the physical samples needed to create datasets and models. Jen’s work uses machine learning to incorporate prior scientific knowledge into models, allowing for more accurate results despite a small sample size by providing the computational model with more background information than what is included in the samples themselves.
In addition to increasing model performance, Jen is also researching how to better understand and predict disorders of the gut microbiome, like C. difficile infection. By analyzing metabolites in fecal samples instead of just microbes sequencing, her models aim to return more accurate predictions and highlight microbial-metabolic relationships that may be influencing patient outcomes.
“Usually, work with the microbiome involves just looking at the relative abundances of the microbes present,” Jen explains. “We’re utilizing a different method that analyzes the metabolites in the gut. Metabolomics are tied more directly to how the microbiome is functioning and interacting with the host.”
With an influx of new methods, it is also important to pay attention to how the model is making predictions. Making sure that the machine learning is producing results that clinicians are able to interpret—and that researchers and clinicians are in sync—is essential to improving trust in the lab models and informing future research.
HST, Jen says, is the perfect environment for that kind of connective, collaborative work. Small cohort sizes mean that many students stay close to their peers, keeping up a personal network of people with diverse research experiences in a collaborative and supportive environment.