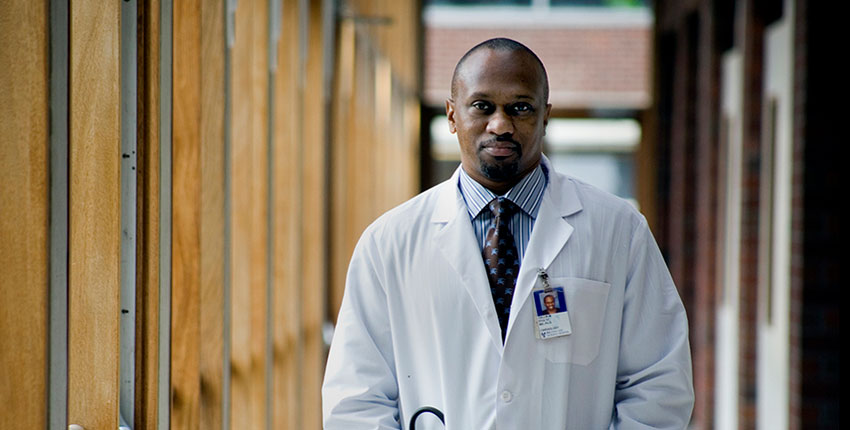
Collin Stultz. Image: M. Scott Brauer
A new HST artificial intelligence in healthcare course bridges domains
Chuck Leddy | IMES
Artificial intelligence is transforming how work gets done across multiple domains, including higher education and healthcare. That transformation is exemplified by a new Harvard-MIT program in Health Sciences and Technology (HST) course called “Artificial Intelligence in Healthcare,” a requirement for first year HST MD students which offers a grounding in the foundations of AI, including the technology’s opportunities and risks.
“If you look at the trajectory of healthcare over the last five to ten years, so much of it has involved the application of artificial intelligence in an effort to improve patient care,” says Collin M. Stultz, Nina T. and Robert H. Rubin Professor, Electrical Engineering & Computer Science, and an associate director, Institute for Medical Engineering & Science (IMES), who teaches the course. Stultz is also the MIT Director of the Harvard-MIT program in Health Sciences and Technology (HST). HST is an inter-institutional collaboration between Harvard University, Harvard Medical School (HMS) and MIT, dedicated to fostering academic excellence, scientific rigor and clinical expertise—IMES is HST's home at MIT.
The next generation of healthcare professionals “will need to be bilingual,” conversant in both computational methods and in the needs of patients in clinical settings, says Vivek F. Farias, the Patrick J. McGovern (1959) Professor at the MIT Sloan School, and an AI researcher. “We need a new generation of talent that’s bilingual across many different domains, such as medical students who appreciate computer science and computer scientists who appreciate the context of healthcare,” he says.
Learning the Basics, Tackling Problems
The new course has two parts, one in August and one in January. In August, students start with a series of didactic lectures and problem sets which expose them to fundamental topics in AI. They then dissect papers on AI applications in healthcare during presentations. “The goal of the August class,” says Stultz, HST MD '97, “is to get students literate enough so that they can read the literature in this space through a critical lens.”
In January, students choose a healthcare-related problem which they’re passionate about, and then work alongside a computer science graduate student to achieve a solution. “The computer scientist helps guide the students in crafting a solution using methods grounded in artificial intelligence and machine learning,” says Stultz.
Becoming Bilingual
There's no requirement that the medical student enrolled in “Artificial Intelligence in Healthcare” have any background in computer science. Students learn to train deep learning models that address clinically meaningful problems and rigorously evaluate their performance. In the process, they learn a new language, a technical vernacular to discuss the opportunities and limits of AI and machine learning in healthcare.
“Getting them all on a similar footing, given their different backgrounds with computational methods, is the most challenging part of [teaching] the course,” says Stultz. “So, when students work together on projects, we try to include at least one ‘computational’ individual in each group.”
Helping Physicians “Look” Deeper
Physicians have a plethora of data available to them—medical records, images, physiologic signals, lab tests, and more—“but they simply cannot process all of it,” says Stultz. AI’s value is that it can leverage massive amounts of data and computational power that no human being can possibly match.
Stultz offers an example. “When humans look at an image, they don't analyze and closely examine every single pixel. We just look and say ‘that's a cat or a child,’ but we necessarily lose some information, those granular details, along the way.”
AI can “uncover subtle relationships in the data that are difficult for even a master clinician to deduce,” says Stultz.
Impactful AI Applications
There are a growing number of AI applications currently impacting healthcare. “One is cancer diagnosis and prognosis,” says Stultz, “where there’s groundbreaking work around using medical images, such as routine screening for breast cancer and lung cancer, for determining who will eventually develop cancer.”
Another noteworthy application is “using wearable devices in order to predict who will get atrial fibrillation,” says Stultz.
As more AI applications evolve within healthcare, medical professionals will not only need to understand their use in clinical settings, but must also develop the ability to explain AI-generated results and methods to patients.
As first year medical student Zoe Weiss, a first-year HST MD-PhD student, whois enrolled in the course, explains, “my medical school classmates and I could be involved in future decisions about implementing AI in hospitals, so learning the fundamentals of how AI works will help us shape how it’s used for the benefit of our patients.”
The Explainability Challenge
A lot of machine learning systems are so-called “black boxes,” meaning humans can’t understand or explain precisely how a result was generated.
Explainability is a bigger concern in healthcare than in “traditional” computer science settings, says Stultz. “Computer scientists who don't work in the area of medicine will sometimes say that explainability isn’t so important,” he says, “but when you speak to clinicians and patients, they unambiguously say that explainability is highly important.”
Clinicians must be able to explain the computational methods used to reach a result, know what the benefits and risks are to patients, and “have the ability to communicate all of that in a manner that engenders trust that they’re doing things in the best interest of patients,” says Stultz.
The “Biased Data” Challenge
Data sets used to train computational models often reflect the biases within humans. “The algorithms we develop inherit our biases. This is not a nefarious statement, but just a statement of fact demonstrated by countless studies,” says Stultz. “Bias [in AI] can be very nuanced and very challenging to decipher.”
Andrew Castillo, also first-year HST MD-PhD student, has developed ways to evaluate computational models for bias: “the course has taught me to ask critical questions to ensure patient safety. Has a model been tested for bias? Do I anticipate it will work for the patient in front of me? What are the risks if the model is wrong? These key questions help ensure that AI actually improves patient care rather than unintentionally harms it.”
Angelo Kayser-Browne, HST MD ’28, agrees: “only by acknowledging that a model can perpetuate the biases of the society that created it can we begin to ensure that AI is geared toward breaking down systems of discrimination rather than continuing them.”
A New Discipline
“Artificial intelligence as applied to healthcare is a new scientific discipline that continues to evolve,” says Stultz. “The best learning experiences within this new discipline occur when you bring together individuals who speak different languages [computer science and patient care]. The resulting ‘information transfer’ leads to something new that benefits healthcare providers, computer scientists, and ultimately patients.”
Kayser-Browne offers a final word: “Innovation in the world of AI and healthcare obviously demands technical expertise, but it also requires a humanistic vision and the wisdom to ask the ‘right’ questions. It’s important to remember that the purpose of these models is to help human beings in a practical way.”